- Artificial Intelligence (AI) in Agriculture Market Analy-sis by Size, Share, Growth, Trends up to 2025
New 2019 Report on “Artificial In-telligence (AI) in Agriculture Market size | Industry Segment by Applications (Precision Farming, Livestock Monitoring, Drone Analytics, Agriculture Robots and Others), by Type (Machine Learning, Computer Vision and Predictive Analytics), Regional Outlook, Market Demand, Latest Trends, Arti-ficial Intelligence (AI) in Agriculture Industry Share & Revenue by Manufacturers, Company Profiles, Growth Forecasts – 2025.” Analyzes current market size and upcoming 5 years growth of this industry. The recent report on the Artificial Intelligence (AI) in Agriculture market is a docu-mentation of the end-to-end study of this industry, and includes crucial information about the business vertical, taking into account key factors such as the current market trends, profit predic-tions, market size, market share, and periodic deliverables across the projected timeline. A con-cise outline of the Artificial Intelligence (AI) in Agriculture market in terms of defining parameters over the assessment period has been given in the report. Additionally, details about the key pro-pellers shaping the market dynamics and influencing the growth rate which the industry will wit-ness over the analysis period have been detailed. Also, the Artificial Intelligence (AI) in Agriculture market study provides a crisp understanding of the challenges which will command this business sphere, in conjunction with the growth opportunities present. Request Sample Copy of this Report @ https://www.zzreport.com/request-sample/650 - Artificial Intelligence (AI) in Drug Discovery Market 2020 Updated & COVID 19 Outbreak Impact Analysis | Microsoft Cor-poration, NVIDIA Corporation, IBM Corporation, Google Inc.
The ‘ Artificial Intelligence (AI) in Drug Discovery market’ research report added by Report Ocean, is an in-depth analysis of the lat-est developments, market size, status, upcoming technologies, industry drivers, challenges, regu-latory policies, with key company profiles and strategies of players. The research study provides market overview, Artificial Intelligence (AI) in Drug Discovery market definition, regional market opportunity, sales and revenue by region, manufacturing cost analysis, Industrial Chain, market ef-fect factors analysis, Artificial Intelligence (AI) in Drug Discovery market size forecast, market da-ta & Graphs and Statistics, Tables, Bar & Pie Charts, and many more for business intel-ligence. Global Artificial Intelligence (AI) in Drug Discovery Market valued approximately USD XX million in 2016 is anticipated to grow with a healthy growth rate of more than XX% over the fore-cast period 2017-2025. In-depth information by Market Size, competitive landscape is provided i.e. Revenue (Million USD) by Players (2013-2018), Revenue Market Share (%) by Players (2013-2018) and further a qualitative analysis is made towards market concentration rate, product/service dif-ferences, new entrants and the technological trends in future. This is a latest report, covering the current COVID-19 impact on the market. The pandemic of Coronavirus (COVID-19) has affected every aspect of life globally. This has brought along several changes in market conditions. The rap-idly changing market scenario and initial and future assessment of the impact is covered in the report. Experts have studied the historical data and compared it with the changing market situa-tions. The report covers all the necessary information required by new entrants as well as the ex-isting players to gain deeper insight. Download Free Sample Copy of ‘ Artificial Intelligence (AI) in Drug Discovery market’ Report @ https://www.reportocean.com/industry-verticals/sample-request?report_id=bw790 - Artificial Intelligence Business Models
Perpetual License, Cloud-Based, On-Premises, Pre-Built Solutions, Embedded Solutions, and Hybrid Pricing Models for AI Soft-ware - Artificial Intelligence for Breast MRI in 2008–2018: A Systematic Mapping Review, Marina Codari, Simone Schiaffino, Francesco Sardanelli, and Rubina Manuela Trimboli
OBJECTIVE. The purpose of this study is to review literature from the past decade on applications of artificial intelligence (AI) to breast MRI. MATERIALS AND METHODS. In June 2018, a systematic search of the literature was performed to identify articles on the use of AI in breast MRI. For each article identified, the sur-name of the first author, year of publication, journal of publication, Web of Science Core Collec-tion journal category, country of affiliation of the first author, study design, dataset, study aim(s), AI methods used, and, when available, diagnostic performance were recorded. RESULTS. Sixty-seven studies, 58 (87%) of which had a retrospective design, were analyzed. When journal catego-ries were considered, 36% of articles were identified as being included in the radiology and imag-ing journal category. Contrast-enhanced sequences were used for most AI applications (n = 50; 75%) and, on occasion, were combined with other MRI sequences (n = 8; 12%). Four main clinical aims were addressed: breast lesion classification (n = 36; 54%), image processing (n = 14; 21%), prognostic imaging (n = 9; 13%), and response to neoadjuvant therapy (n = 8; 12%). Artificial neu-ral networks, support vector machines, and clustering were the most frequently used algorithms, accounting for 66%. The performance achieved and the most frequently used techniques were then analyzed according to specific clinical aims. Supervised learning algorithms were primarily used for lesion characterization, with the AUC value from ROC analysis ranging from 0.74 to 0.98 (median, 0.87) and with that from prognostic imaging ranging from 0.62 to 0.88 (median, 0.80), whereas unsupervised learning was mainly used for image processing purposes. CONCLUSION. In-terest in the application of advanced AI methods to breast MRI is growing worldwide. Although this growth is encouraging, the current performance of AI applications in breast MRI means that such applications are still far from being incorporated into clinical practice. - Artificial Intelligence for Medical Image Analysis: A Guide for Authors and Review-ers, Joseph R. England and Phillip M. Cheng
OBJECTIVE. The purpose of this article is to highlight best practices for writing and reviewing articles on artificial intelligence for medical image analysis. CONCLUSION. Artificial intelligence is in the early phases of application to medical imaging, and patient safety demands a commitment to sound methods and avoidance of rhetorical and overly optimistic claims. Adherence to best practices should elevate the quality of articles submitted to and published by clinical journals. - Artificial Intelligence in Agriculture Market Worth $4.0 Billion by 2026 – Exclusive Report by MarketsandMarkets
According to the new market re-search report “Artificial Intelligence in Agriculture Market by Technology (Machine Learning, Com-puter Vision, and Predictive Analytics), Offering (Software, Hardware, AI-as-a-Service, and Ser-vices), Application, and Geography – Global Forecast to 2026”, published by MarketsandMarkets™, the Artificial Intelligence in Agriculture Market is estimated to be USD 1.0 billion in 2020 and is projected to reach USD 4.0 billion by 2026, at a CAGR of 25.5% between 2020 and 2026. The mar-ket growth is driven by the increasing implementation of data generation through sensors and aerial images for crops, increasing crop productivity through deep-learning technology, and gov-ernment support for the adoption of modern agricultural techniques. Request for PDF Brochure: https://www.marketsandmarkets.com/pdfdownloadNew.asp?id=159957009 - Artificial Intelligence in Breast Imaging: Potentials and Limitations, Ellen B. Men-delson
OBJECTIVE. The purpose of this article is to discuss potential applications of artificial intelligence (AI) in breast imaging and limi-tations that may slow or prevent its adoption. CONCLUSION. The algorithms of AI for workflow improvement and outcome analyses are advancing. Using imaging data of high quality and quan-tity, AI can support breast imagers in diagnosis and patient management, but AI cannot yet be re-lied on or be responsible for physicians’ decisions that may affect survival. Education in AI is ur-gently needed for physicians. - Artificial Intelligence in Cardiothoracic Radiology, William F. Auffermann1, Elliott K. Gozansky and Srini Tridandapani
OBJECTIVE. The goal of this article is to examine some of the current cardiothoracic radiology applications of artificial intelligence in general and deep learning in particular. CONCLUSION. Artificial intelligence has been used for the analysis of medical images for decades. Recent advances in computer algorithms and hardware, coupled with the availability of larger labeled datasets, have brought about rapid advances in this field. Many of the more notable recent advances have been in the artificial intelligence subfield of deep learning. - Artificial Intelligence in China: Landscape Analysis of Key AI Technologies, Arnold Gao, Arun Chandrasekaran, 2020
AI is an important area of invest-ment in China, with a rapidly evolving ecosystem. Enterprise architecture and technology innova-tion leaders should stay abreast of emerging technologies being developed and assess the oppor-tunities and risks from megavendors’ strategies and startups in that market - Artificial Intelligence in IoT Market Outlook, Recent Trends and Growth Forecast 2020-2025
The Analysis report titled “Artifi-cial Intelligence in IoT Market 2025” highly demonstrates the current Artificial Intelligence in IoT market analysis scenario, impending future opportunities, revenue growth, pricing and profitabil-ity of the industry. Growth Analysis Report on “Artificial Intelligence in IoT Market size | Industry Segment by Applications (Small & Medium Scale Business and Large Scale Busines), by Type (On-Premise and Cloud-based), Regional Outlook, Market Demand, Latest Trends, Artificial Intelli-gence in IoT Industry Share & Revenue by Manufacturers, Company Profiles, Growth Fore-casts – 2025.” Analyzes current market size and upcoming 5 years growth of this industry. Re-quest Sample Copy of this Report @ https://www.zzreport.com/request-sample/2254 Artificial Intelligence in IoT Market report delivers the close outlook of top companies with their strategies, growth factors, Artificial Intelligence in IoT industry analysis by region and so on. Also, this report is analyzed based on the Key Stakeholders, Downstream Vendors, Distributors, Traders and new entrants in the Artificial Intelligence in IoT Market. Manufacturer / Potential Investors, Traders, Distributors, Wholesalers, Retailers, Importers and Exporters, Association and government bodies are the main audience for Artificial Intelligence in IoT market involved in this report. - Artificial Intelligence in Mar-keting 2020-2027 Growth Analysis and Forecast
Artificial Intelligence in Marketing Industry 2020 Global Market Analysis report gives the In-depth analysis of historical data along with size, share, growth, demand, revenue and forecast of the global Artificial Intelligence in Marketing and estimates the future trend of market on the basis of this detailed study. The report shares market performance both in terms of volume and revenue and this factor which is useful & helpful to the business. To get sample Copy of the report, along with the TOC, Statistics, and Tables please visit @ https://www.theinsightpartners.com/sample/TIPRE00002954/ - Artificial Intelligence in Musculoskeletal Imaging: Current Status and Future Direc-tions, Soterios Gyftopoulos, Dana Lin, Florian Knoll, Ankur M. Doshi …
OBJECTIVE. The objective of this article is to show how artificial intelligence (AI) has impacted different components of the imag-ing value chain thus far as well as to describe its potential future uses. CONCLUSION. The use of AI has the potential to greatly enhance every component of the imaging value chain. From as-sessing the appropriateness of imaging orders to helping predict patients at risk for fracture, AI can increase the value that musculoskeletal imagers provide to their patients and to referring cli-nicians by improving image quality, patient centricity, imaging efficiency, and diagnostic accura-cy. - Artificial Intelligence in Precision Medicine Market Professional Market, Top key players – Intel AI,IBM,GE Healthcare,Thermo Fisher Scien-tific Post author, accounts@reportsandmarkets.com, 2020
Global Artificial Intelligence in Precision Medicine Market Professional Market 2020 Research Report The Global Artificial Intelli-gence in Precision Medicine Market Professional Market 2020 Research Report is a professional and in-depth study on the current state of Artificial Intelligence in Precision Medicine Market Pro-fessional Market. The key manufacturers covered in this report are: Intel AI,IBM,GE Healthcare,Thermo Fisher Scientific,Qiagen,Berg Health,Medasense Biometrics. The final report will add the analysis of the Impact of Covid-19 in this report Cell-Free DNA (cfDNA) Testing indus-try. - Artificial Intelligence in Video Games Mar-ket Size by Top Key Players, Growth Opportunities, Incremental Revenue , Outlook and Forecasts to 2026
Global Artificial Intelligence in Video Games Market 2020: This is a latest report, covering the current COVID-19 impact analysis on the market. This has led to several changes in market conditions. The rapidly changing market scenario as well as the first and future impact assessment are covered with in the report. The Ar-tificial Intelligence in Video Games Market research report included analysis of various factors that increase market growth. It contains trends, restrictions and drivers that change the market positively or negatively. The Artificial Intelligence in Video Games Market Report includes all key factors that affect global and regional markets, including drivers, detention, threats, challenges, risk factors, opportunities, and industry trends. This business research paper provides an in-depth assessment of all critical aspects of the global market in relation to Artificial Intelligence in Video Games market size, market share, market growth factor, main suppliers, sales, value, volume, main regions, industry trends, product demand, capacity, cost structure and Artificial Intelligence in Video Games market expansion. The report begins with an overview of the structure of the in-dustry chain and describes the industry environment. Then the size of the market and the Artificial Intelligence in Video Games forecasts are analyzed by product type, application, end use and re-gion. The report presents the situation of competition on the market between suppliers and the profile of the company. In addition, this report analyzes the market prices and treated the charac-teristics of the value chain. For Better Understanding, Download Free Sample Copy Of Artificial Intelligence in Video Games Market Report @ https://www.marketresearchintellect.com/download-sample/?rid=194013&utm_source=LHN&utm_medium=888 - Artificial Intelligence Maturity Mode, Svetlana Sicular, Bern Elliot, Whit Andrews, Pieter den Hamer, 2020
Artificial intelligence technologies impact most application categories and many business challenges. Data and analytics leaders can use our AI maturity model to accelerate and optimize their AI strategy and implementations to achieve the best value from AI technologies - Artificial Intelligence Platform Market Research, Recent Trends and Growth Forecast 2025
Latest Market Research Report on “Artificial Intelligence Platform Market size | Industry Segment by Applications (Voice Processing, Text Processing and Image Processing), by Type (On-Premise and Cloud-based), Regional Outlook, Market Demand, Latest Trends, Artificial Intelligence Platform Industry Share & Revenue by Manufacturers, Company Profiles, Growth Forecasts – 2025.” Analyzes current market size and upcoming 5 years growth of this industry. The report on Artificial Intelligence Platform market is an all-inclusive study of the current scenario of the industry and its growth prospects over 2025. The report is a meticulous endeavor to present a comprehensive overview of Artificial Intelligence Platform market based on growth opportunities and market shares. The report presents a detailed outline of the product type, key manufacturers, application and key regions concerned in the Arti-ficial Intelligence Platform market. Request Sample Copy of this Report @ https://www.zzreport.com/request-sample/2152 This report considers various parameters to cal-culate the Artificial Intelligence Platform market size especially, value and volume generated from the sales in such segments as product type, application, region, competitive landscape etc. - Artificial Intelligence Software Industry Size 2019, Market Opportunities, Share Analysis up to 2025
Latest Market Research Report on “Artificial Intelligence Software Market size | Industry Segment by Applications (Voice Processing, Text Processing and Image Processing), by Type (On-Premise and Cloud-based), Regional Outlook, Market Demand, Latest Trends, Artificial Intelligence Software Industry Share & Revenue by Manufacturers, Company Profiles, Growth Forecasts – 2025.” Analyzes current market size and upcoming 5 years growth of this industry. The research report of Artificial Intelligence Software market is predicted to accrue a significant renumeration portfolio by the end of the predicted time period. It includes parameters with respect to the Artificial Intelligence Software market dynam-ics – incorporating varied driving forces affecting the commercialization graph of this business vertical and risks prevailing in the sphere. In addition, it also speaks about the Artificial Intelli-gence Software Market growth opportunities in the industry. Request Sample Copy of this Report @ https://www.zzreport.com/request-sample/2101 Artificial Intelligence Software Market Re-port covers the manufacturers’ data, including shipment, price, revenue, gross profit, interview record, business distribution etc., these data help the consumer know about the competitors bet-ter. This report also covers all the regions and countries of the world, which shows a regional de-velopment status, including Artificial Intelligence Software market size, volume and value, as well as price data. - Artificial Intelligence Software System Market Forecast 2020-2025, Latest Trends and Opportunities
Latest Market Research Report on “Artificial Intelligence Software System Market size | Industry Segment by Applications (Voice Processing, Text Processing and Image Processing), by Type (On-Premise and Cloud-based), Re-gional Outlook, Market Demand, Latest Trends, Artificial Intelligence Software System Industry Share & Revenue by Manufacturers, Company Profiles, Growth Forecasts – 2025.” Analyzes current market size and upcoming 5 years growth of this industry. As per the report, the Artificial Intelligence Software System market is predicted to gain significant returns while registering a lucrative annual growth rate during the foreseen time period. Exposing an enthralling outline of this Artificial Intelligence Software System industry, the report provides details about the com-plete valuation of the market, growth opportunities in the business verticals along with a detailed classification of the Artificial Intelligence Software System market. Request Sample Copy of this Report @ https://www.zzreport.com/request-sample/2046 Artificial Intelligence Software Sys-tem Market report is an extensive analysis of all available companies with their growth factors, research & methodology, Artificial Intelligence Software System Market Dynamics, Business Overview, Sales, Revenue, Artificial Intelligence Software System Market Share and Competition with other Manufacturers. - Artificial Intel-ligence Systems in Healthcare Market Share Analysis and Research Report by 2025
New 2019 Report on “Artificial In-telligence Systems in Healthcare Market size | Industry Segment by Applications (Hospitals, Am-bulatory Surgery Centers, Clinics and Others), by Type (On-Premise and Cloud-Based), Regional Outlook, Market Demand, Latest Trends, Artificial Intelligence Systems in Healthcare Industry Share & Revenue by Manufacturers, Company Profiles, Growth Forecasts – 2025.” Analyzes current market size and upcoming 5 years growth of this industry. The report on Artificial Intelli-gence Systems in Healthcare market is an all-inclusive study of the current scenario of the indus-try and its growth prospects over 2025. The report is a meticulous endeavor to present a compre-hensive overview of Artificial Intelligence Systems in Healthcare market based on growth oppor-tunities and market shares. The report presents a detailed outline of the product type, key manu-facturers, application and key regions concerned in the Artificial Intelligence Systems in Healthcare market. Request Sample Copy of this Report @ https://www.zzreport.com/request-sample/1895 This report considers various parameters to calculate the Artificial Intelligence Sys-tems in Healthcare market size especially, value and volume generated from the sales in such segments as product type, application, region, competitive landscape etc. - Artificial Intelligence Trends: Decision Augmentation, Procurement Research Team, 2020
Organizations report that AI, es-pecially related to decision augmentation, has high potential to influence corporate decision mak-ing. We provide a comprehensive review for procurement leaders of recent use cases, technology and adoption trends related to applications of AI in decision augmentation - Assessing DevOps in Artificial Intelligence Initiatives, Carlton Sapp, 2020
Organizations increasingly rely on rapidly delivering and productionizing AI to achieve goals. DevOps plays a significant role in shap-ing how data and analytics technical professionals deploy AI. This research assesses the practice of DevOps in AI initiatives - Augmented Analytics Market Analysis, Trends, Forecast, 2018 – 2025, Sameer Joshi, 2020
Augmented analytics embeds ma-chine learning algorithms, natural language generation, and other advanced analytics functionality into business intelligence (BI) to automate insights. Augmented analytics comprises data prepara-tion, data discovery, and augmented data science and machine learning (ML). Augmented analyt-ics utilizes automated ML to transform how data is developed, consumed, and shared. Non-technical users can easily interface with augmented analytics solutions by asking questions direct-ly and getting answers instantly, radically decreasing reporting time, and accelerating strategy and performance. The growing adoption of it will enable organizations to optimize decisions and actions of not only data scientists but also all employees. To get sample Copy of the report, along with the TOC, Statistics, and Tables please visit @: https://www.premiummarketinsights.com/sample/AMR00014108 Rise in need to democratize the analytics and increase productivity, increase in awareness of enterprises to utilize growing streams of data from various sources in innovative ways, and to make the work of citizen data scientists and business users easier are some of the major factors that drive the growth of the global augmented analytics market. In addition, adoption of modern business intelligence tools by enterprises, which utilize artificial intelligence algorithms and machine learning is expected to fuel the growth of the market. Leading Players in the Augmented Analytics Market: IBM Corpora-tion, Qlik, Tableau Software, Tibco Software, Salesforce, Sisense Inc., SAP SE, SAS Institute, Mi-crosoft, and ThoughtSpot. - Augmented Analytics Market Exclusive Report by 2027 | IBM Corpo, Microsoft Cor-po, Oracle Corpo, QlikTech International, SAP SE, SAS Post author, Sameer Joshi, 2020
The latest market intelligence study on Augmented Analytics relies on the statistics derived from both primary and secondary re-search to present insights pertaining to the forecasting model, opportunities, and competitive landscape of Augmented Analytics market for the forecast period. Using the powers of machine learning for automating data insights fir enabling good visualizations of data to the end-user is defined as augmented analytics. Augmented analytics enables scientists and data analysts to formulate various strategies on different business aspects. Accessible augmented analytics cre-ates citizen data scientists and improves accountability and empowerment. These solutions pro-duce better decisions, more accurate business predictions and measurable analysis of product and service offerings, pricing, financials, production and other aspects of business. - Augmented Analytics Market Lat-est Report with Forecast to 2029
The rising need and importance of data along with the evolution of next-generation technologies and data processing tools is gener-ating potential opportunities for the organization’s business models and operations. The demand for augmented analytics is increasing continuously among organizations to streamline business processes and to cater the need for advanced data processing tools and solutions. Moreover, augmented reality also offered an edge over the traditional analysis tools by providing clear in-formation and by automating data insights. Get Sample Copy of the Report @ https://www.tmrresearch.com/sample/sample?flag=B&rep_id=6468 - Augmented and Virtual Reality in Healthcare Market Major Drivers and Challenges by: Google, Atheer, Psious, Microsoft, Medical Realities, reportsintellect, 2020
The ultra-modern research Aug-mented and Virtual Reality in Healthcare Market each qualitative and quantitative records analy-sis to provide an overview of the destiny adjacency around Augmented and Virtual Reality in Healthcare Market for the forecast duration, 2020-2025. The Augmented and Virtual Reality in Healthcare Market’s boom and developments are studied and an in depth review is been given. Get Sample Copy of this Report at https://www.reportsintellect.com/sample-request/957123 A thorough examine of the competitive panorama of the Augmented and Virtual Reality in Healthcare Market has been supply imparting insights into the enterprise profiles, economic re-pute, latest traits, mergers and acquisitions, and the SWOT analysis. It gives a cultured view of the classifications, packages, segmentations, specifications and lots of extra for Augmented and Virtual Reality in Healthcare Market. This market studies is an intelligence record with meticulous efforts undertaken to take a look at the right and treasured data. Regulatory situations that have an effect on the diverse decisions in the Augmented and Virtual Reality in Healthcare Market are given a keen statement and have been explained. - Augmented and Virtual Reality Software and Service Market Top Key Players Analysis till 2024 by: Mozilla, Google, Apple, Pixar, Facebook
Augmented and Virtual Reality Software and Service Market each qualitative and quantitative records analysis to provide an overview of the destiny adjacency around Augmented and Virtual Reality Software and Service Market for the forecast duration, 2019-2024. The Augmented and Virtual Reality Software and Service Market’s boom and developments are studied and an in depth review is been given. Get Sample Copy of this Report at https://www.reportsintellect.com/sample-request/1024189 - Augmented Intelligence Market 2020: Current Trend, Demand, Scope, Business Strategies, Technology Development, Future Investment And Forecast 2025
The global augmented intelli-gence market report [5 Years Forecast 2020-2025] focuses on the COVID19 Outbreak Impact anal-ysis of key points influencing the growth of the market. Providing info like market competitive sit-uation, product scope, market overview, opportunities, driving force and market risks. The report has analyzed revenue impact of covid-19 pandemic on the sales revenue of augmented intelli-gence market leaders, market followers and disrupters in the report and same is reflected in our analysis. Top Company Profile covers: SparkCognition, Microsoft Cognitive services, Numenta and IBM Watson among several others Get Sample PDF (including COVID19 Impact Analysis, full TOC, Tables and Figures) at: https://www.adroitmarketresearch.com/contacts/request-sample/769 - Augmented Intelligence Market Analysis and Demand with Forecast Overview To 2025
This research articulation on augmented intelligence market is a thorough collation of crucial primary and secondary research postulates. This augmented intelligence market also harps on competitive landscape, accurately identifying and assessing market forerunners in the augmented intelligence market and their growth rendering initiatives. This thought provoking intricately crafted perspective of the aug-mented intelligence market is aimed at offering unfailing cues on market growth as a composite whole that aim at presenting all the nitty gritty of the market to encourage unfaltering growth scope despite stringent competition in the augmented intelligence market. Top leading players of the market are: SparkCognition, Microsoft Cognitive services, Numenta and IBM Watson among several others Get Sample Copy of this Report: https://www.adroitmarketresearch.com/contacts/request-sample/769 - Augmented Intelligence Market Size, Share, Analysis, Applications, Sale, Growth Insight, Trends, Leaders, Services and 2025 Forecast
Adroit market research anticipate the Augmented Intelligence Market to be among the fastest-growing sectors worldwide in the coming years. The augmented intelligence Market is broadly studied by the authors of the report with large focus on the vendor landscape, regional expansion, leading segments, rising trends and key opportunities, and other important subjects. The report highlights powerful factors augment-ing the demand in the augmented intelligence market and even those hampering the global mar-ket growth - Augmented Reality and Virtual Reality in Aerospace Market Research 2020 by: Vuzix, Epson, Google Inc., Microsoft, Magic Leap Inc, Kopin Corporation
Augmented Reality and Virtual Reality in Aerospace Market research report displays the market size, share, status, production, cost analysis, and market value with the forecast period 2020-2025. The overall analysis of Ad-vanced Augmented Reality and Virtual Reality in Aerospace Market covers an overview of the in-dustry policies. The report also details the information about the top key players, sales, revenue, future trends, research findings, and opportunities. The prime objective of this report is to help the user understand the Augmented Reality and Virtual Reality in Aerospace Market in terms of its definition, segmentation, market potential, influential trends, and the challenges that the mar-ket is facing. Get Sample Copy of this Report at https://www.reportsintellect.com/sample-request/1072161?ata Some of the leading market players: Vuzix, Epson, Google Inc., Microsoft, Magic Leap Inc, Kopin Corporation - Business Analytics and Artificial Intelligence for Technical Professionals Primer for 2020, Carlton Sapp, 2020
Analytics and AI techniques, driv-en by advances in machine learning, are rapidly evolving to support digital businesses at scale. Technical professionals must mature analytics capabilities with modern solutions and architec-tures to accelerate delivery - Can Texture Analysis Be Used to Distinguish Benign From Malignant Adrenal Nod-ules on Unenhanced CT, Contrast-Enhanced CT, or In-Phase and Opposed-Phase MRI?, Lisa M. Ho, Ehsan Samei, Maciej A. Mazurowski, Yuese Zheng …
OBJECTIVE. The purpose of this study is to determine whether second-order texture analysis can be used to distinguish lipid-poor adenomas from malignant adrenal nodules on unenhanced CT, contrast-enhanced CT (CECT), and chemical-shift MRI. MATERIALS AND METHODS. In this retrospective study, 23 adrenal nodules (15 lipid-poor adenomas and eight adrenal malignancies) in 20 patients (nine female patients and 11 male patients; mean age, 59 years [range, 15–80 years]) were assessed. All patients under-went unenhanced CT, CECT, and chemical-shift MRI. Twenty-one second-order texture features from the gray-level cooccurrence matrix and gray-level run-length matrix were calculated in 3D. The mean values for 21 texture features and four imaging features (lesion size, unenhanced CT attenuation, CECT attenuation, and signal intensity index) were compared using a t test. The diag-nostic performance of texture analysis versus imaging features was also compared using AUC values. Multivariate logistic regression models to predict malignancy were constructed for texture analysis and imaging features. RESULTS. Lesion size, unenhanced CT attenuation, and the signal intensity index showed significant differences between benign and malignant adrenal nodules. No significant difference was seen for CECT attenuation. Eighteen of 21 CECT texture features and nine of 21 unenhanced CT texture features revealed significant differences between benign and malignant adrenal nodules. CECT texture features (mean AUC value, 0.80) performed better than CECT attenuation (mean AUC value, 0.60). Multivariate logistic regression models showed that CECT texture features, chemical-shift MRI texture features, and imaging features were predictive of malignancy. CONCLUSION. Texture analysis has a potential role in distinguishing benign from malignant adrenal nodules on CECT and may decrease the need for additional imaging studies in the workup of incidentally discovered adrenal nodules. - Chronic Obstructive Pulmonary Disease Quantification Using CT Texture Analysis and Densitometry: Results From the Danish Lung Cancer Screening, Trial Lauge Sørensen, Mads Nielsen, Jens Petersen, Jesper H. Pedersen …
OBJECTIVE. The purpose of this study is to establish whether texture analysis and densitometry are complementary quantitative measures of chronic obstructive pulmonary disease (COPD) in a lung cancer screening setting. MATERIALS AND METHODS. This was a retrospective study of data collected prospectively (in 2004–2010) in the Danish Lung Cancer Screening Trial. The texture score, relative area of emphy-sema, and percentile density were computed for 1915 baseline low-dose lung CT scans and were evaluated, both individually and in combination, for associations with lung function (i.e., forced expiratory volume in 1 second as a percentage of predicted normal [FEV1% predicted]), diagnosis of mild to severe COPD, and prediction of a rapid decline in lung function. Multivariate linear re-gression models with lung function as the outcome were compared using the likelihood ratio test or the Vuong test, and AUC values for diagnostic and prognostic capabilities were compared using the DeLong test. RESULTS. Texture showed a significantly stronger association with lung function (p < 0.001 vs densitometric measures), a significantly higher diagnostic AUC value (for COPD, 0.696; for Global Initiative for Chronic Obstructive Lung Disease (GOLD) grade 1, 0.648; for GOLD grade 2, 0.768; and for GOLD grade 3, 0.944; p < 0.001 vs densitometric measures), and a high-er but not significantly different association with lung function decline. In addition, only texture could predict a rapid decline in lung function (AUC value, 0.538; p < 0.05 vs random guessing). The combination of texture and both densitometric measures strengthened the association with lung function and decline in lung function (p < 0.001 and p < 0.05, respectively, vs texture) but did not improve diagnostic or prognostic performance. CONCLUSION. The present study highlights texture as a promising quantitative CT measure of COPD to use alongside, or even instead of, den-sitometric measures. Moreover, texture may allow early detection of COPD in subjects who un-dergo lung cancer screening. - Combat Digital Transformation Fatigue by Applying a Neuroscience Finding, Daniel Sanchez Reina, Mary Mesaglio, 2020
Digital transformation can cause change fatigue in organizations, putting the initiative at risk of failure, due to too much change or change for nothing. CIOs can use a neuroscience finding to mitigate that risk - Comparison of Artificial Intelligence–Based Fully Automatic Chest CT Emphysema Quantification to Pulmonary Function Testing,Andreas M. Fischer, Akos Varga-Szemes, Marly van Assen, L. Parkwood Griffith …
OBJECTIVE. The purpose of this study was to evaluate an artificial intelligence (AI)-based prototype algorithm for fully automated quantification of emphysema on chest CT compared with pulmonary function testing (spirometry). MATERIALS AND METHODS. A total of 141 patients (72 women, mean age ± SD of 66.46 ± 9.7 years [range, 23–86 years]; 69 men, mean age of 66.72 ± 11.4 years [range, 27–91 years]) who underwent both chest CT acquisition and spirometry within 6 months were retrospectively includ-ed. The spirometry-based Tiffeneau index (TI; calculated as the ratio of forced expiratory volume in the first second to forced vital capacity) was used to measure emphysema severity; a value less than 0.7 was considered to indicate airway obstruction. Segmentation of the lung based on two different reconstruction methods was carried out by using a deep convolution image-to-image network. This multilayer convolutional neural network was combined with multilevel feature chaining and depth monitoring. To discriminate the output of the network from ground truth, an adversarial network was used during training. Emphysema was quantified using spatial filtering and attenuation-based thresholds. Emphysema quantification and TI were compared using the Spearman correlation coefficient. RESULTS. The mean TI for all patients was 0.57 ± 0.13. The mean percentages of emphysema using reconstruction methods 1 and 2 were 9.96% ± 11.87% and 8.04% ± 10.32%, respectively. AI-based emphysema quantification showed very strong corre-lation with TI (reconstruction method 1, ρ = −0.86; reconstruction method 2, ρ = −0.85; both p < 0.0001), indicating that AI-based emphysema quantification meaningfully reflects clinical pulmo-nary physiology. CONCLUSION. AI-based, fully automated emphysema quantification shows good correlation with TI, potentially contributing to an image-based diagnosis and quantification of emphysema severity. - Critical Capabilities for Data Science and Machine Learning Platforms, Pieter den Hamer, Alexander Linden, Carlie Idoine, Erick Brethenoux, Jim Hare, Svetlana Sicular, Farhan Choudhary, Peter Kerensky, 2020
The functions and features of data science and machine learning platforms are evolving quickly to keep pace with a highly innovative space. This research helps data and analytics leaders to evaluate 16 of these platforms across 15 critical capabilities - Data Engineering for Machine Learning in Women’s Imaging and Beyond, Chen Cui, Shinn-Huey S. Chou, Laura Brattain, Constance D. Lehman …
OBJECTIVE. Data engineering is the foundation of effective machine learning model development and research. The accuracy and clinical utility of machine learning models fundamentally depend on the quality of the data used for model development. This article aims to provide radiologists and radiology researchers with an understanding of the core elements of data preparation for machine learning research. We cover key concepts from an engineering perspective, including databases, data integrity, and char-acteristics of data suitable for machine learning projects, and from a clinical perspective, includ-ing the HIPAA, patient consent, avoidance of bias, and ethical concerns related to the potential to magnify health disparities. The focus of this article is women’s imaging; nonetheless, the princi-ples described apply to all domains of medical imaging. CONCLUSION. Machine learning research is inherently interdisciplinary: effective collaboration is critical for success. In medical imaging, radiologists possess knowledge essential for data engineers to develop useful datasets for ma-chine learning model development. - Deep Learning Market Growth Opportunities, Challenges, Key Companies, Drivers and Forecast to 2026
Global Deep Learning Market 2020: This is a latest report, covering the current COVID-19 impact analysis on the market. This has led to several changes in market conditions. The rapidly changing market scenario as well as the first and future impact assessment are covered with in the report. The Deep Learning Market research report included analysis of various factors that increase market growth. It contains trends, restrictions and drivers that change the market positively or negatively. The Deep Learning Market Report includes all key factors that affect global and regional markets, including drivers, detention, threats, challenges, risk factors, opportunities, and industry trends. This business re-search paper provides an in-depth assessment of all critical aspects of the global market in rela-tion to Deep Learning market size, market share, market growth factor, main suppliers, sales, val-ue, volume, main regions, industry trends, product demand, capacity, cost structure and Deep Learning market expansion. The report begins with an overview of the structure of the industry chain and describes the industry environment. Then the size of the market and the Deep Learning forecasts are analyzed by product type, application, end use and region. The report presents the situation of competition on the market between suppliers and the profile of the company. In addi-tion, this report analyzes the market prices and treated the characteristics of the value chain. For Better Understanding, Download Free Sample Copy Of Deep Learning Market Report @ https://www.verifiedmarketresearch.com/download-sample/?rid=6905&utm_source=COD&utm_medium=005 - Deep Learning Model to Assess Cancer Risk on the Basis of a Breast MR Image Alone, Tally Portnoi, Adam Yala, Tal Schuster, Regina Barzilay …
OBJECTIVE. The purpose of this study is to develop an image-based deep learning (DL) model to predict the 5-year risk of breast cancer on the basis of a single breast MR image from a screening examination. MATERIALS AND METHODS. We collected 1656 consecutive breast MR images from screening examinations per-formed for 1183 high-risk women from January 2011 to June 2013, to predict the risk of cancer developing within 5 years of the screening. Women who lacked a 5-year screening follow-up ex-amination and women who had cancer other than primary breast cancer develop in their breast were excluded from the study. We developed a logistic regression model based on traditional risk factors (the risk factor logistic regression [RF-LR] model) and a DL model based on the MR image alone (the Image-DL model). Examinations occurring within 6 months of a cancer diagnosis were excluded from the testing sets in each fold of cross-validation. We compared our models against the Tyrer-Cuzick (TC) model. All models were evaluated using mean (± SD) AUC values and ob-served-to-expected (OE) ratios across 10-fold cross-validation. RESULTS. The RF-LR and Image-DL models achieved mean AUC values of 0.558 ± 0.108 and 0.638 ± 0.094, respectively. In contrast, the TC model achieved an AUC value of 0.493 ± 0.092. The Image-DL and RF-LR models achieved mean OE ratios of 0.993 ± 0.658 and 0.828 ± 0.181, compared with the mean OE ratio of 1.091 ± 0.255 obtained using the TC model. CONCLUSION. Our DL model can assess the 5-year cancer risk on the basis of a breast MR image alone, and it showed improved individual risk discrimination when compared with a state-of-the-art risk assessment model. These results offer promising pre-liminary data regarding the potential of image-based risk assessment models to support more personalized care. - Deep Learning Soft-ware Market Expected to Witness the Highest Growth 2025 with Top Key Players Microsoft, Google, IBM, Amazon Web Services, etc
Reports Monitor has added a re-port titled, “Global Deep Learning Software Market Professional Report 2020-2025” into its data-base of research report. The study provides complete details about the usage and adoption of Deep Learning Software in various industrial applications and geographies. This helps the key stakeholders in knowing about the major development trends, growth strategies, investments, vendor activities, and government initiatives. Moreover, the report specifies the major drivers, re-straints, challenges, and lucrative opportunities that are going to impact the growth of the mar-ket. The Top Leading players operating in the market: Covered in this Report: Microsoft, Google, IBM, Amazon Web Services, Nuance Communications, NCH Software, Clarifai, GitHub, BigHand, TRINT, NVIDIA, Sight Machine, Alibaba, Hive, Harris Geospatial Solutions, SAS Institute, IMC & More. To Download PDF Sample Report, With 30 mins free consultation! Click Here: https://www.reportsmonitor.com/request_sample/408386 - Demystifying Artificial Intelligence: AI in Total Rewards and Performance Manage-ment, 2020
Artificial intelligence is growing rapidly in prevalence, but total rewards leaders struggle to understand what it can do for their function. This presentation details four use cases for AI in total rewards and performance man-agement, and steps to prepare for implementation - Effect of CT Reconstruction Algorithm on the Diagnostic Performance of Radiomics Models: A Task-Based Approach for Pulmonary Subsoil Nodules, Hyungjin Kim, Chang Min Park, Jeonghwan Gwak, Eui Jin Hwang …
OBJECTIVE. We investigated whether the diagnostic performance of machine learning–based radiomics models for the dis-crimination of invasive pulmonary adenocarcinomas (IPAs) among subsolid nodules (SSNs) was affected by the proportion of images reconstructed with filtered back projection (FBP) and model-based iterative reconstruction (MBIR) in datasets used for feature extraction. MATERIALS AND METHODS. This retrospective study included 60 patients (23 men and 37 women; mean age, 61.4 years) with 69 SSNs (54 part-solid and 15 pure ground-glass nodules). Preoperative CT scans were reconstructed with both FBP and MBIR. A total of 860 radiomics features were obtained from the entire nodule volume, and 70 resampled nodule datasets with an increasing proportion of nodules with MBIR-derived features (from 0/69 to 69/69) were prepared. After feature selection using neighborhood component analysis, support vector machines (SVMs) and an ensemble model were used as classifiers for the differentiation of IPAs. The diagnostic performances of all blending pro-portions of reconstruction algorithms were calculated and analyzed. RESULTS. The ROC AUC and the diagnostic accuracy of the radiomics models decreased significantly as the number of nodules with MBIR-derived features increased, and this relationship followed cubic functions (R2 = 0.993 and 0.926 for SVM; R2 = 0.993 and 0.975 for the ensemble model; p < 0.001). The magnitude of variation in AUC due to the reconstruction algorithm heterogeneity was 0.39 for SVM and 0.39 for the ensemble model. CONCLUSION. Inclusion of CT scans reconstructed with MBIR for radiomics modeling can significantly decrease diagnostic performance for the identification of IPAs. - Emerging Technologies and Trends Impact Radar: Artificial Intelli-gence, Annette Jump, Eric Goodness, Nick Ingelbrecht, Alys Woodward, Anthony Bradley, 2019
How far artificial intelligence technologies and techniques are from early mainstream adoption is a crucial reference point for planning product portfolios and roadmaps. Therefore, product managers must understand the timing and potential impact of AI technologies across market segments and sectors - exture Analysis of Imaging: What Radiologists Need to Know, Bino A. Varghese, Steven Y. Cen, Darryl H. Hwang and Vinay A. Duddalwar
OBJECTIVE. Radiologic texture is the variation in image intensities within an image and is an important part of radiomics. The ob-jective of this article is to discuss some parameters that affect the performance of texture met-rics and propose recommendations that can guide both the design and evaluation of future radi-omics studies. CONCLUSION. A variety of texture-extraction techniques are used to assess clinical imaging data. Currently, no consensus exists regarding workflow, including acquisition, extraction, or reporting of variable settings leading to poor reproducibility. - Gartner Sur-vey Reveals Leading Organizations Expect to Double the Number of AI Projects In Place Within the Next Year, Stamford Conn., 2019
Improved Customer Experience and Task Automation Are Key Drivers of AI Use - Getting Real About AI, Data Science and Machine Learning: Gartner Talks, Austin Kronz – Peter Kerensky, 2020
Discussion Topics: What is the role of governance and regulation in the age of AI; Which technologies will best enable your data science and machine learning initiatives; What is the role of citizen data scientists. So many ques-tions still surround artificial intelligence (AI), data science and machine learning. Yet, the ques-tions are getting far less theoretical as more organizations look to take advantage of these tech-nologies now. Organizations are asking more about how they can apply these technologies, how long it will take to gain the benefits, and how to train staff — or if they need to hire new staff. Join us for this complimentary video webinar as Gartner experts Austin Kronz and Peter Krensky address the most pressing questions asked directly by your peers - Global Artificial Intelligence (AI) In Cybersecurity Market, Analysis, Drivers, Restraints, Opportunities, Threats, Trends, Applica-tions, And Forecast To 2029, 2020
- Global Artificial Intelligence (AI) in Transportation Market -Industry Analysis and Forecast (2020-2027)
Global Artificial Intelligence (AI) in Transportation Market was valued at US$ XX Bn in 2019 and is expected to reach US$ XX Bn by 2027, at a CAGR of XX % during a forecast period.The report study has analyzed revenue impact of covid-19 pandemic on the sales revenue of market leaders, market followers and disrupters in the report and same is reflected in our analysis. Major driving factors of the Artificial Intelligence (AI) in Transportation Market are the transportation problems are the rising system behaviour with difficult to model according to a predictable pattern, affecting by things like traffic, human errors, or accidents. REQUEST FOR FREE SAMPLE REPORT: https://www.maximizemarketresearch.com/request-sample/24592 - Global Artificial Intelligence (AI) Market: Investments vs Potential, 2020
The scope of this report is broad and covers the global markets for artificial intelligence, which is increasingly being implemented across a wide range of industries for various applications.The market is broken down by solution, end-user industry, technology and region. Revenue forecasts from 2019 to 2024 are presented for each type, technology, end-user industry, and regional market. The report also includes a discus-sion of the major players in each regional market for artificial intelligence.It explains the major market drivers of the global market, current trends in the industry and the regional dynamics of the artificial intelligence market. The report concludes with detailed profiles of major vendors in the global artificial intelligence industry - Global Artificial Intelligence for Defense – Market and Technology Forecast to 2028, 2020
Artificial Intelligence (AI) is a fast-developing field of technology with possibly substantial implications for national security. Defense departments of countries spanning the US, European Union, Russia, and China are financing the development of AI applications for a range of military functions. AI research is underway in the fields of intelligence collection and analysis, logistics, cyber operations, information operations, command and control, and in a variety of semi-autonomous and autonomous vehicles. Already, AI has been incorporated into military operations in Iraq and Syria - Global Artificial Intelligence in Oil and Gas Market 2020 Growing Demand, Latest Trends and Developments, Growth Analysis Till 2025
The study is based on the estima-tion of the trends, which are based on the present, future and the strategies which are used in the past. It also determines and estimates the views and opinions which are expressed by the con-sumers. These are used for the prediction and analysis of the market for the estimated forecast period. This report emphases on consumption, market share and growth rate of Global Artificial Intelligence in Oil and Gas Market. The Global Artificial Intelligence in Oil and Gas Market report supplies business outlining, requirements, contact information and product image of important manufacturers of Global Artificial Intelligence in Oil and Gas Market. Request a sample of this re-port @ https://www.orbisresearch.com/contacts/request-sample/4586181 The study also pro-vides detailed analysis of the market, which consists of the growth of the regions, which is one of the major aspects which is likely to have an impact on the market. The strengths and the political factors, which are likely to affect the market is also covered in detail for the estimation of the market in the estimated forecast. Moreover, increased demand for the growth of the products in the specific market is also one of the major attributes which are likely to have an impact on the growth of the market in the estimated forecast period. Key vendors/manufacturers in the market: IBM (US), Numenta (US), Accenture (Republic of Ireland), Intel (US), Oracle (US), Microsoft (US), Inbenta (US), NVIDIA Corporation(US), Google (US), Sentient technologies (US), Hortonworks (US), General, Vision (US), Royal Dutch Shell (Netherlands), Infosys (India), Cisco (US), FuGenX Technologies (US) Browse the complete report @ https://www.orbisresearch.com/reports/index/global-artificial-intelligence-in-oil-and-gas-market-2020-by-key-countries-companies-type-and-application - Global Artificial Intelligence In The Telecommunication Market Size & Fore-cast, Market Research Intellect, 2020
Nel rapporto, i ricercatori hanno fornito un’analisi quantitativa e qualitativa e una valutazione delle opportunità - Global Augmented Analyt-ics Market : Industry Analysis and Forecast (2020-2027)
Global Augmented Analytics Mar-ket was valued US$ 6.28 Bn in 2019 and is expected to reach US$ 41.38 Bn by 2027 at a CAGR of 30.9 %. This report provides a detailed analysis of the market segment based on insurance type, sales channel and region. This report also focuses on the top players in North America, Europe, Asia Pacific, Middle East & Africa, and South America. The report study has analyzed revenue impact of covid-19 pandemic on the sales revenue of market leaders, market followers and dis-rupters in the report and same is reflected in our analysis. REQUEST FOR FREE SAMPLE REPORT: https://www.maximizemarketresearch.com/request-sample//27122/ - Global aug-mented analytics market 2020 (includes business impact of covid-19)
Trusted Business Insights answers what are the scenarios for growth and recovery and whether there will be any lasting structural impact from the unfolding crisis for the Augmented Analytics Market market. Trusted Business In-sights presents an updated and Latest Study on Augmented Analytics Market Market 2019-2026. The report contains market predictions related to market size, revenue, production, CAGR, Con-sumption, gross margin, price, and other substantial factors. While emphasizing the key driving and restraining forces for this market, the report also offers a complete study of the future trends and developments of the market. The report further elaborates on the micro and macroeconomic aspects including the socio-political landscape that is anticipated to shape the demand of the Augmented Analytics Market market during the forecast period (2019-2029). It also examines the role of the leading market players involved in the industry including their corporate overview, fi-nancial summary, and SWOT analysis. Get Sample Copy of this Report https://www.trustedbusinessinsights.com/details/global-augmented-analytics-market-2020 - Global Deep Learning Chip Market Growth (Status and Outlook) 2020-2026
A research report on the Global Deep Learning Chip Market delivers complete analysis regarding the size, trends, market share, and growth prospects. In addition, the report includes market volume with an exact opinion of-fered in the report. This research report assesses the market growth rate and the industry value depending on the growth such as driving factors, market dynamics, and other associated data. The information provided in this report is integrated based on the trends, latest industry news, as well as opportunities. The Deep Learning Chip market report is major compilation of major information with respect to the overall competitor data of this market. Likewise, the information is an inclu-sive of the number of regions where the global Deep Learning Chip industry has fruitfully gained the position. This research report delivers a broad assessment of the Deep Learning Chip market. The global Deep Learning Chip market report is prepared with the detailed verifiable projections, and historical data about the Deep Learning Chip market size. Request a sample of this report @ https://www.orbisresearch.com/contacts/request-sample/4421459 - Global Emotion Artificial Intelligence Market Size, Status and Forecast 2020-2026, 2020
Emotion AI is a new field that analysis of a person’s verbal and non-verbal communication in order to understand the person’s mood or attitude, then can be used in CRM (Customer Relationship Management) area, such as to identify how a customer perceives a product, the presentation of a product or an interaction with a company representative. Market Analysis and Insights: Global Emotion Artificial Intelligence Market In 2019, the global Emotion Artificial Intelligence market size was US$ xx million and it is expected to reach US$ xx million by the end of 2026, with a CAGR of xx% during 2021-2026 - Global Machine Learning as a Ser-vice Market Size, Status and Forecast 2020-2026, Precision Report 2020
Machine learning is a field of arti-ficial intelligence that uses statistical techniques to give computer systems the ability to “learn” (e.g., progressively improve performance on a specific task) from data, without being explicitly programmed. Market Analysis and Insights: Global Machine Learning as a Service Market In 2019, the global Machine Learning as a Service market size was US$ 1715.2 million and it is expected to reach US$ 6871.1 million by the end of 2026, with a CAGR of 21.8% during 2021-2026 - Global Machine Learning Market 2020-2024 | Increasing Adoption of Cloud-Based Offerings to Boost the Market Growth | Technavio, 2020
The rising adoption of cloud com-puting services globally is increasing the adoption of cloud-based applications aimed at multiple end-user industries. The inherent benefits of cloud computing, such as minimal cost for compu-ting, network and storage infrastructure, scalability, reliability, and high resource availability, en-courage enterprises to adopt cloud-based solutions in their business models. Machine learning adopted via the cloud enables enterprises to experiment with machine learning technologies and capabilities at a fraction of the cost of setting up an in-house machine learning team and infra-structure. Machine learning also helps enterprises to scale up the production workload of their projects with the increase in data. The above-mentioned advantages of cloud-based offerings are expected to drive the growth of the global machine learning market - Global Operational Intelligence Market Overview, Orbit Research, 2020
1 INTRODUCTION: 1.1 Study De-liverables, 1.2 Study Assumptions, 1.3 Scope of the Study, 2 RESEARCH METHODOLOGY, 3 EXEC-UTIVE SUMMARY, 4 MARKET DYNAMICS: 4.1 Market Overview, 4.2 Introduction to Market Drivers and Restraints, 4.3 Market Drivers, 4.3.1 Need for Real Time Data Analytics, 4.4 Market Restraints 4.4.1 Combining Data from Multiple Data Sources is Challenging the Market, 4.5 Industry Attrac-tiveness – Porter’s Five Force Analysis, 4.5.1 Threat of New Entrants, 4.5.2 Bargaining Power of Buyers/Consumers, 4.5.3 Bargaining Power of Suppliers, 4.5.4 Threat of Substitute Products, 4.5.5 Intensity of Competitive Rivalry, 5 MARKET SEGMENTATION, 5.1 By Deployment Type: 5.1.1 On-Premise, 5.1.2 Cloud, 5.2 By End-user Vertical, 5.2.1 Retail, 5.2.2 Manufacturing, 5.2.3 Financial Services, 5.2.4 Government, 5.2.5 IT & Telecommunication, 5.2.6 Military & Defense, 5.2.7 Transport & Logistics, 5.2.8 Healthcare, 5.2.9 Energy & Power, 5.3:Geography: 5.3.1 North America, 5.3.2 Europe, 5.3.3 Asia-Pacific, 5.3.4 Latin America 5.3.5 Middle East & Africa, 6 COMPETITIVE LANDSCAPE, 6.1 Company Profiles: 6.1.1 Vitria Technology Inc., 6.1.2 Splunk Inc., 6.1.3 Starview Inc., 6.1.4 SAP SE, 6.1.5 Software AG, 6.1.6 Schneider Electric, 6.1.7 Rolta India Limited, 6.1.8 SolutionsPT Ltd, 6.1.9 IBENOX Pty Ltd., 6.1.10 Turnberry Corporation, 6.1.11 HP Inc., 6.1.12 OpenText Corp., 7 INVESTMENT ANALYSIS, 8, MARKET OPPORTUNITIES AND FUTURE TRENDS - Global trade impact of the coronavirus signals intelligence (sigint) market aug-mented expansion to be registered by 2019-2033
he Signals Intelligence (SIGINT) market research encompasses an exhaustive analysis of the market outlook, framework, and socio-economic impacts. The report covers the accurate investigation of the market size, share, product footprint, revenue, and progress rate. Driven by primary and secondary researches, the Signals In-telligence (SIGINT) market study offers reliable and authentic projections regarding the technical jargon.All the players running in the global Signals Intelligence (SIGINT) market are elaborated thoroughly in the Signals Intelligence (SIGINT) market report on the basis of proprietary technolo-gies, distribution channels, industrial penetration, manufacturing processes, and revenue. In addi-tion, the report examines R&D developments, legal policies, and strategies defining the competitiveness of the Signals Intelligence (SIGINT) market players.The report on the Signals In-telligence (SIGINT) market provides a birdâs eye view of the current proceeding within the Si-gnals Intelligence (SIGINT) market. Further, the report also takes into account the impact of the novel COVID-19 pandemic on the Signals Intelligence (SIGINT) market and offers a clear assess-ment of the projected market fluctuations during the forecast period. Get Free Sample PDF (in-cluding COVID19 Impact Analysis, full TOC, Tables and Figures) of Market Report @ https://www.researchmoz.com/enquiry.php?type=S&repid=2601770&source=atm - Global Trend in Artificial Intelligence–Based Publications in Radiology From 2000 to 2018, Elizabeth West, Simukayi Mutasa, Zelos Zhu and Richard Ha
OBJECTIVE. The purpose of this study is to evaluate the global trend in artificial intelligence (AI)-based research productivity invol-ving radiology and its subspecialty disciplines. CONCLUSION. The United States is the global lea-der in AI radiology publication productivity, accounting for almost half of total radiology AI output. Other countries have increased their productivity. Notably, China has increased its productivity ex-ponentially to close to 20% of all AI publications. The top three most productive radiology subspe-cialties were neuroradiology, body and chest, and nuclear medicine. - GPU for Deep Learning Market Outlook 2020 Witnessing Enormous Growth with Recent Trends & Demand | Nvidia, AMD, Intel
The research report contains a de-tailed summary of the Global GPU for Deep Learning Market that includes various well-known or-ganizations, manufacturers, vendors, key market players who are leading in terms of revenue ge-neration, sales, dynamic market changes, end-user demands, products and services offered, re-stricted elements in the market, products and other processes. Technical advancements, market bifurcation, surplus capacity in the developing GPU for Deep Learning markets, globalization, re-gulations, production and packaging are some of the factors covered in this report. The research report on Global GPU for Deep Learning Market is a detailed study of the current market scenario, covering the key market trends and dynamics. The report also presents a logical evaluation of the major challenges faced by the leading market players operating in the market, which helps the participants to understand the barriers and challenges they may face in future while functioning in the international market over the forecast 2019-2025. The novel COVID-19 pandemic has put the world on a standstill, affecting major operations, leading to an industrial catastrophe. This report presented by Garner Insights contains a thorough analysis of the pre and post pandemic market scenarios. This report covers all the recent development and changes recorded during the COVID-19 outbreak. Get a PDF Sample Copy (including COVID19 Impact Analysis, TOC, Tables, and Figu-res) @ https://garnerinsights.com/Global-GPU-for-Deep-Learning-Market-Professional-Survey-Report-2019#request-sample - Healthcare Artificial Intelli-gence Market Research Report, Growth Forecast 2025
Growth Forecast Report on “Heal-thcare Artificial Intelligence Market size | Industry Segment by Applications (Patient Data and Risk Analysis, Lifestyle Management and Monitoring, Precision Medicine, In-Patient Care and Ho-spital Management, Medical Imaging and Diagnosis and Other), by Type (Hardware, Software and Services), Regional Outlook, Market Demand, Latest Trends, Healthcare Artificial Intelligence In-dustry Share & Revenue by Manufacturers, Company Profiles, Growth Forecasts – 2025.” Analyzes current market size and upcoming 5 years growth of this industry. The latest research report on the Healthcare Artificial Intelligence market is an in-depth documentation of this mar-ket space and entails detailed summary of various market segmentations. The report summarized the market sphere and provides gist of the Healthcare Artificial Intelligence market with regards to the industry size as well as current position, on the basis of volume and revenue. The study fur-ther entails information pertaining to the regional scope of the market, alongside the key compa-nies operating in the competitive landscape of the Healthcare Artificial Intelligence market. The Healthcare Artificial Intelligence market report consist competitive study of the major Healthcare Artificial Intelligence manufacturers which will help to develop a marketing strategy. Request Sample Copy of this Report @ https://www.zzreport.com/request-sample/224 - How Cognitive Machines Can Augment Medical Imaging, D. Douglas Miller, and Eric W. Brown
OBJECTIVE. Artificial intelligence (AI) neural networks rapidly convert disparate facts and data into highly predictive analytic mo-dels. Machine learning maps image-patient phenotype correlations opaque to standard statistics. Deep learning performs accurate image-derived tissue characterization and can generate virtual CT images from MRI datasets. Natural language processing reads medical literature and efficient-ly reconfigures years of PACS and electronic medical record information. CONCLUSION. AI logistics solve radiology informatics workflow pain points. Imaging professionals and companies will drive health care AI technology insertion. Data science and computer science will jointly potentiate the impact of AI applications for medical imaging. - How to Choose the Right AI Solution Path: From Off-the-Shelf to Custom-Made, Alexander Linden, Eric Hunter, Farhan Choudhary, Peter Kerensky, 2020
For each AI use case, data and analytics leaders must determine which type of solution will best fit their current constraints and needs. Gartner provides a summary of the pros and cons of various solution paths to aid in making this decision - Impact of Artificial Intelligence on Women’s Imaging: Cost-Benefit Analysis, Ray C. Mayo and Jessica W. T. Leung
OBJECTIVE. The purpose of this article is to identify and discuss four areas in which artificial intelligence (AI) must excel to beco-me clinically viable: performance, time, work flow, and cost. CONCLUSION. AI holds tremendous potential for transforming the practice of radiology, but certain metrics are needed to objectively quantify its impact. As patients, physicians, hospitals, and insurance companies look for value, AI must earn a role in medical imaging. - Indagine conoscitiva sui Big Data, AGCM, AGCOM, Garante per la priva-cy
Premessa, 1. I Big, Data: 1.1; In-troduzione ai Big Data; 1.2. Definizioni;1.3. La filiera deiBig Data; 1.3.1. La raccolta dei Big Data; 1.3.2. L’elaborazione dei Big Data; 1.3.3. L’interpretazione e l’utilizzo dei Big Data; 1.4. Alcuni dati sulla diffusione dell’utilizzo dei Big Data nell’economia, 2.Principali considerazioni sulla gestione dei Big Data espresse dai soggetti partecipanti: 2.1. Profilazione, anonimizzazione del dato e algo-ritmi; 2.2. Gestione del dato e acquisizione del consenso; 2.3. Portabilità dei dati, interoperabilità e accesso ai dati; 2.4. Utilizzo dei dati di traffico; 2.5. Piattaforme digitali: pluralismo dell’informazione e potere di mercato, 3. I Big Data nell’ecosistema digitale italiano: considera-zioni dell’AGCOM: 3.1; Big Data, mercato pubblicitario, pluralismo e informazione; 3.2. Big Data, comunicazioni elettroniche e servizi media; 3.3. Big Data e sviluppo di reti e servizi innovativi (5G, IoT, M2M, AI); Big Data e altri settori; 3.5. Big Data ed evoluzione del quadro regolamentare eu-ropeo, 4. I Big Data nell’ecosistema digitale italiano: considerazioni del Garante per la protezione dei dati personali: 4.1. Premessa; 4.2. Gli interventi dei soggetti istituzionali; 4.3. Oltre la pura de-scrizione del fenomeno; 4.4. Le implicazioni etich; 4.5. Big Data, principio di qualità dei dati (e dei processi) e profilazione; 4.6. Per un approccio win-win; 4.7. L’opacità dei trattamenti con tecniche Big Data e il principio di trasparenza proprio delle discipline di protezione dei dati; 4.8. Big Data, dati personali e procedure di anonimizzazione; 4.9. Big Data e principio di finalità; 4.10. Big Data, principi di qualità e minimizzazione dei dati; Big Data, valutazione d’impatto privacy e accountabi-lity; 4.12. Big Data e processi decisionali automatizzati; 4.13. Big Data e grandi archivi pubblici; 4.14. Prospettive, 5. I Big Data nell’ecosistema digitale italiano: considerazioni dell’AGCM; 5.1. Big Data, struttura di mercato e barriere all’entrata; 5.2. Posizioni dominanti e potere di mercato; 5.3. Big Data, utilizzo dei dati personali e concorrenza; 5.3.1. Premessa; 5.3.2. L’acquisizione di dati personali nel processo produttivo e benessere del consumatore; 5.3.3. La raccolta e l’utilizzo dei dati personali come variabile economica; 5.3.4. La relazione tra concorrenza e utilizzo dei dati personali; 5.3.5. Domanda e offerta di dati personali; 5.3.6. Privacy, funzionamento dei mercati e il ruolo della politica pubblica; 5.4. Condotte data-driven tra la tutela della concorrenza e la tutela del consumatore; 5.4.1. La raccolta di dati; 5.4.2. L’utilizzo dei Big Data per la personalizzazione dei servizi; 5.4.3. L’utilizzo dei Big Data per la personalizzazione dei prezzi; 5.4.4. Condotte che possono integrare possibili abusi di posizione dominante; 5.4.5. L’utilizzo di Big Data, algoritmi di prezzo e cllusione online, LINEE GUIDA E RACCOMANDAZIONI DI POLICY - Intelligenza Artificiale per le PMI, Steering Committee Digitalizzazione PMI – Confindustria digitale, 2019
Capitolo 1: Sfruttare l’Intelligenza Artificiale come potente strumento di competitività; Definizione; Ambiti, Capitolo 2: Gli investi-menti delle PMI in competenze e nuove modalità di lavoro; Cosa cambia con l’intelligenza artifi-ciale?.; Nuove figure professionali e competenze; Capitolo 3 L’Intelligenza Artificiale come parte integrante della trasformazione digitale; I dati al centro dell’evoluzione tecnologica; Progettazione e strategia imprenditoriale; Approccio tattico; Approccio strategico, Capitolo 4: Casi concreti di uti-lizzo dell’Intelligenza Artificiale in ambito industriale; I dati al centro del nuovo modello organiz-zativo; Le persone, la nostra forza lavoro; I processi, la nostra spina dorsale; I clienti, i nostri mi-gliori alleati; I prodotti, la nostra eccellenza; Il Customer Service, per completare l’eccellenza del prodotto, Capitolo 5 Intelligenza Artificiale come servizio; Se l’Intelligenza artificiale fosse una uti-lity; IA come servizio: AIaaS è solo una parte del contesto; Come utilizzare l’IA tramite servizi; IA come servizio: considerazioni finali, Prossimi Passi - Interpretable Artificial Intelligence: Why and When, Adarsh Ghosh and Devasena-thipathy Kandasamy
OBJECTIVE. The purpose of this article is to discuss the problem of interpretability of artificial intelligence (AI) and highlight the need for continuing scientific discovery using AI algorithms to deal with medical big data. CON-CLUSION. A plethora of AI algorithms are currently being used in medical research, but the opacity of these algorithms makes their clinical implementation a dilemma. Clinical decision making can-not be assigned to something that we do not understand. Therefore, AI research should not be li-mited to reporting accuracy and sensitivity but, rather, should try to explain the underlying reasons for the predictions, in an attempt to enrich biologic understanding and knowledge. - Invest Implications: ‘Predicts 2020: Artificial Intelligence Core Technologies’, Tim Mahon, 2019
Enterprises struggle to develop AI pilots into production, limiting their ability to realize AI’s business value. Investors benefit from understanding how, and with what, vendors help IT leaders nurture strategies that enable the evolution of AI pilots into scalable production and value realization - Julia Lan-guage in Machine Learning: Algorithms, Applications, and Open Issues, Kaifeng Gao, Jingzhi Tu, Zenan Huo, Gang Mei, Francesco Piccialli, Salvatore Cuomo, 2020
Machine learning is driving deve-lopment across many fields in science and engineering. A simple and efficient programming lan-guage could accelerate applications of machine learning in various fields. Currently, the pro-gramming languages most commonly used to develop machine learning algorithms include Py-thon, MATLAB, and C/C ++. However, none of these languages well balance both efficiency and simplicity. The Julia language is a fast, easy-to-use, and open-source programming language that was originally designed for high-performance computing, which can well balance the efficiency and simplicity. This paper summarizes the related research work and developments in the appli-cation of the Julia language in machine learning. It first surveys the popular machine learning al-gorithms that are developed in the Julia language. Then, it investigates applications of the machi-ne learning algorithms implemented with the Julia language. Finally, it discusses the open issues and the potential future directions that arise in the use of the Julia language in machine lear-ning - LATEST MARKET RESEARCH REPORT ON DEEP LEAR-NING CHIPSET | CONSUMER, AEROSPACE, MILITARY & DEFENSE, AUTOMOTIVE, INDU-STRIAL, MEDICAL
The report provides the market fo-recast of Deep Learning Chipset market for atleast next 5 years which would help investors, indu-stry analyst and strategist to take informed decisions while creating Deep Learning Chipset busi-ness strategies. The Deep Learning Chipset market report contains industry top manufacturers di-scussion based on the company’s profiles, financial analysis, overview, market revenue, and op-portunities by geographical regions. Get Free Sample Report and Related Graphs & Charts @: https://www.marketintellica.com/report/MI26181-global-deep-learning-chipset-market-status#enquir This study consists of market segmentation by Deep Learning Chipset product types, applications and Deep Learning Chipset market division based on geographical regions : USA, Eu-rope, China, India, Southeast Asia, Japan, South America, South Africa and Others - Machine learning and artificial intelligence research for patient benefit: 20 critical questions on transparency, replicability, ethics, and effectiveness, 2020
Machine learning, artificial intelli-gence, and other modern statistical methods are providing new opportunities to operationalise previously untapped and rapidly growing sources of data for patient benefit. Despite much promi-sing research currently being undertaken, particularly in imaging, the literature as a whole lacks transparency, clear reporting to facilitate replicability, exploration for potential ethical concerns, and clear demonstrations of effectiveness. Among the many reasons why these problems exist, one of the most important (for which we provide a preliminary solution here) is the current lack of best practice guidance specific to machine learning and artificial intelligence. However, we belie-ve that interdisciplinary groups pursuing research and impact projects involving machine learning and artificial intelligence for health would benefit from explicitly addressing a series of questions concerning transparency, reproducibility, ethics, and effectiveness (TREE). The 20 critical que-stions proposed here provide a framework for research groups to inform the design, conduct, and reporting; for editors and peer reviewers to evaluate contributions to the literature; and for pa-tients, clinicians and policy makers to critically appraise where new findings may deliver patient benefit - Machine Learning for the Interventional Radiologist, Ryan D. Meek, Matthew P. Lungren and Judy W. Gichoya
OBJECTIVE. The purpose of this article is to describe key potential areas of application of machine learning in interventional radio-logy. CONCLUSION. Machine learning, although in the early stages of development within the field of interventional radiology, has great potential to influence key areas such as image analysis, clinical predictive modeling, and trainee education. A proactive approach from current interven-tional radiologists and trainees is needed to shape future directions for machine learning and arti-ficial intelligence. - Machine Learning in Neurooncology Imaging: From Study Request to Diagnosis and Treatment, Javier E. Villanueva-Meyer, Peter Chang, Janine M. Lu-po, Christopher P. Hess …
OBJECTIVE. Machine learning has potential to play a key role across a variety of medical imaging applications. This review seeks to elucidate the ways in which machine learning can aid and enhance diagnosis, treatment, and fol-low-up in neurooncology. CONCLUSION. Given the rapid pace of development in machine learning over the past several years, a basic proficiency of the key tenets and use cases in the field is criti-cal to assessing potential opportunities and challenges of this exciting new technology. - Machine Learning Prediction of Liver Stiffness Using Clinical and T2-Weighted MRI Radiomic Data, Lili He, Hailong Li, Jonathan A. Dudley, Thomas C. Maloney …
OBJECTIVE. The purpose of this study is to develop a machine learning model to categorically classify MR elastography (MRE)–derived liver stiffness using clinical and nonelastographic MRI radiomic features in pediatric and young adult patients with known or suspected liver disease. MATERIALS AND METHODS. Clinical data (27 demographic, anthropomorphic, medical history, and laboratory features), MRI presence of liver fat and chemical shift–encoded fat fraction, and MRE mean liver stiffness measurements were retrieved from electronic medical records. MRI radiomic data (105 features) were extracted from T2-weighted fast spin-echo images. Patients were categorized by mean liver stiffness (< 3 vs ≥ 3 kPa). Support vector machine (SVM) models were used to perform two-class classification using clinical features, radiomic features, and both clinical and radiomic features. Our proposed model was internally evaluated in 225 patients (mean age, 14.1 years) and externally evaluated in an independent cohort of 84 patients (mean age, 13.7 years). Diagnostic performance was asses-sed using ROC AUC values. RESULTS. In our internal cross-validation model, the combination of clinical and radiomic features produced the best performance (AUC = 0.84), compared with clini-cal (AUC = 0.77) or radiomic (AUC = 0.70) features alone. Using both clinical and radiomic featu-res, the SVM model was able to correctly classify patients with accuracy of 81.8%, sensitivity of 72.2%, and specificity of 87.0%. In our external validation experiment, this SVM model achieved an accuracy of 75.0%, sensitivity of 63.6%, specificity of 82.4%, and AUC of 0.80. CONCLUSION. An SVM learning model incorporating clinical and T2-weighted radiomic features has fair-to-good diagnostic performance for categorically classifying liver stiffness. - Machine translation of cortical activity to text with an encoder–decoder framework, Joseph G. Makin, David A. Moses & Edward F. Chang , 2020
A decade after speech was first decoded from human brain signals, accuracy and speed remain far below that of natural speech. Here we show how to decode the electrocorticogram with high accuracy and at natural-speech ra-tes. Taking a cue from recent advances in machine translation, we train a recurrent neural net-work to encode each sentence-length sequence of neural activity into an abstract representation, and then to decode this representation, word by word, into an English sentence. For each partici-pant, data consist of several spoken repeats of a set of 30–50 sentences, along with the contem-poraneous signals from ~250 electrodes distributed over peri-Sylvian cortices. Average word error rates across a held-out repeat set are as low as 3%. Finally, we show how decoding with limited data can be improved with transfer learning, by training certain layers of the network under mul-tiple participants’ data. - Mobile Artificial Intelligence (AI) Market, Zion Market Research, 2020
Mobile Artificial Intelligence (AI) Market by Technology Node (5nm-10nm, 11nm-20nm, and Above 20nm), by Application (Came-ras, Smartphones, Vehicles, Robots, AR/VR Devices, and Others), and by End-Use Industry (Con-sumer Electronics, Automotive, Robotics, and Others): Global Industry Perspective, Comprehensive Analysis, and Forecast, 2017 – 2024 - Nature Medicine, Evaluation and accura-te diagnoses of pediatric diseases using artificial intelligence, Huiying Liang, Brian Y. Tsui, […]Huimin Xia, 2019
Artificial intelligence (AI)-based methods have emerged as powerful tools to transform medical care. Although machine learning classifiers (MLCs) have already demonstrated strong performance in image-based diagnoses, ana-lysis of diverse and massive electronic health record (EHR) data remains challenging. Here, we show that MLCs can query EHRs in a manner similar to the hypothetico-deductive reasoning used by physicians and unearth associations that previous statistical methods have not found. Our mo-del applies an automated natural language processing system using deep learning techniques to extract clinically relevant information from EHRs. In total, 101.6 million data points from 1,362,559 pediatric patient visits presenting to a major referral center were analyzed to train and validate the framework. Our model demonstrates high diagnostic accuracy across multiple organ systems and is comparable to experienced pediatricians in diagnosing common childhood disea-ses. Our study provides a proof of concept for implementing an AI-based system as a means to aid physicians in tackling large amounts of data, augmenting diagnostic evaluations, and to provi-de clinical decision support in cases of diagnostic uncertainty or complexity. Although this impact may be most evident in areas where healthcare providers are in relative shortage, the benefits of such an AI system are likely to be universal - NEW CAS RESEARCH PAPER ON DEEP LEARNING FO-RECASTS FOR INDIVIDUAL CLAIMS
The Casualty Actuarial Society re-cently published a new piece of research with potential immediate applications in individual claims forecasting. Part of the new CAS Research Paper series, “Individual Claims Forecasting with Bayesian Mixture Density Networks” is written by Kevin Kuo and introduces an individual claims forecasting framework utilizing Bayesian mixture density networks; the paper outlines a modeling framework that uses a publicly available data simulation tool. - New Frontiers: An Update on Computer-Aided Diagnosis for Breast Imaging in the Age of Artificial Intelligence, Yiming Gao, Krzysztof J. Geras, Alana A. Lewin and Linda Moy
OBJECTIVE. The purpose of this article is to compare traditional versus machine learning–based computer-aided detection (CAD) platforms in breast imaging with a focus on mammography, to underscore limitations of traditio-nal CAD, and to highlight potential solutions in new CAD systems under development for the futu-re. CONCLUSION. CAD development for breast imaging is undergoing a paradigm shift based on vast improvement of computing power and rapid emergence of advanced deep learning algo-rithms, heralding new systems that may hold real potential to improve clinical care. - News report 2019 – The next newsroom: unlocking the power of AI for public service jornalism, Hanna Stjärne, Director General SVT, 2019
In this EBU News Report of 2019, we examine what the fourth major wave of digital transformation means for public service jour-nalism. This new wave, after online, mobile and social, is defined by opportunities and threats of artificial intelligence and data technologies. There is a lot of hype around AI but for those who see the real potential, it may be able to make public service journalism more valuable to the audience and more inspiring to practice. The report provides a comprehensive review of the current thinking on AI and journalism as well as practical case studies, checklists and tool-kits. This report is open to the public, you just need to login to ebu.ch. If you are new to ebu.ch, simply create an account with us and then click on “View interactive version” - otential Impact of COVID-19 on Augmented Reality and Virtual Reality Apps Market Analyzed in a New Intelligence Study
Analysis of the Global Augmented Reality and Virtual Reality Apps Market The report on the global Augmented Reality and Virtual Reality Apps market reveals that the market is expected to grow at a CAGR of ~XX% during the considered forecast period (2019-2029) and estimated to reach a value of ~US$XX by the end of 2029. The latest report is a valuable tool for stakeholders, established market players, emerging players, and other entities to devise effective strategies to combat the impact of COVID-19 Fur-ther, by leveraging the insights enclosed in the report, market players can devise concise, im-pactful, and highly effective growth strategies to solidify their position in the Augmented Reality and Virtual Reality Apps market. Research on the Augmented Reality and Virtual Reality Apps Market Addresses the Following Queries Which end-user is likely to influence the growth of the Augmented Reality and Virtual Reality Apps market? Which regional market has the highest mar-ket attractiveness in 2020? How are consumer trends impacting the operations of market players in the Augmented Reality and Virtual Reality Apps market? Why are market players aiming to expand their presence in region 1? What are the growth prospects of the Augmented Reality and Virtual Reality Apps market in different regions due to the COVID-19? Get Free Sample PDF (in-cluding COVID19 Impact Analysis, full TOC, Tables and Figures) of Market Report @ https://www.marketresearchhub.com/enquiry.php?type=S&repid=2635303&source=atm - Peering Into the Black Box of Artificial Intelligence: Evaluation Metrics of Machine Learning Methods, Guy S. Handelman, Hong Kuan Kok, Ronil V. Chandra, Amir H. Razavi, …
OBJECTIVE. Machine learning (ML) and artificial intelligence (AI) are rapidly becoming the most talked about and controversial topics in radiology and medicine. Over the past few years, the numbers of ML- or AI-focused stu-dies in the literature have increased almost exponentially, and ML has become a hot topic at aca-demic and industry conferences. However, despite the increased awareness of ML as a tool, many medical professionals have a poor understanding of how ML works and how to critically appraise studies and tools that are presented to us. Thus, we present a brief overview of ML, explain the metrics used in ML and how to interpret them, and explain some of the technical jargon associa-ted with the field so that readers with a medical background and basic knowledge of statistics can feel more comfortable when examining ML applications. CONCLUSION. Attention to sample size, overfitting, underfitting, cross validation, as well as a broad knowledge of the metrics of machine learning, can help those with little or no technical knowledge begin to assess machine learning studies. However, transparency in methods and sharing of algorithms is vital to allow clinicians to assess these tools themselves. - Performance of a Deep Learning Algorithm in Detecting Osteonecrosis of the Femo-ral Head on Digital Radiography: A Comparison With Assessments by Radiologists, Choong Guen Chee, Youngjune Kim, Yusuhn Kang, Kyong Joon Lee …
OBJECTIVE. The objective of our study was to compare the sensitivity of a deep learning (DL) algorithm with the assessments by radiologists in diagnosing osteonecrosis of the femoral head (ONFH) using digital radiography. MATERIALS AND METHODS. We performed a two-center, retrospective, noninferiority study of consecutive patients (≥ 16 years old) with a diagnosis of ONFH based on MR images. We investi-gated the following four datasets of unilaterally cropped hip anteroposterior radiographs: training (n = 1346), internal validation (n = 148), temporal external test (n = 148), and geographic external test (n = 250). Diagnostic performance was measured for a DL algorithm, a less experienced ra-diologist, and an experienced radiologist. Noninferiority analyses for sensitivity were performed for the DL algorithm and both radiologists. Subgroup analysis for precollapse and postcollapse ONFH was done. RESULTS. Overall, 1892 hips (1037 diseased and 855 normal) were included. Sensitivity and specificity for the temporal external test set were 84.8% and 91.3% for the DL al-gorithm, 77.6% and 100.0% for the less experienced radiologist, and 82.4% and 100.0% for the experienced radiologist. Sensitivity and specificity for the geographic external test set were 75.2% and 97.2% for the DL algorithm, 77.6% and 75.0% for the less experienced radiologist, and 78.0% and 86.1% for the experienced radiologist. The sensitivity of the DL algorithm was noninferior to that of the assessments by both radiologists. The DL algorithm was more sensitive for precollapse ONFH than the assessment by the less experienced radiologist in the temporal external test set (75.9% vs 57.4%; 95% CI of the difference, 4.5–32.8%). CONCLUSION. The sensitivity of the DL al-gorithm for diagnosing ONFH using digital radiography was noninferior to that of both less expe-rienced and experienced radiologist assessments. - Prediction of Immunohistochemistry of Suspected Thyroid Nodules by Use of Ma-chine Learning–Based Radionics, Jiabing Gu, Jian Zhu, Qingtao Qiu, Yungang Wang …
OBJECTIVE. The purpose of this study was to develop and validate a radiomics model for evaluating immunohistochemical charac-teristics in patients with suspected thyroid nodules. MATERIALS AND METHODS. A total of 103 patients (training cohort–to-validation cohort ratio, ≈ 3:1) with suspected thyroid nodules who had undergone thyroidectomy and immunohistochemical analysis were enrolled. The immunohisto-chemical markers were cytokeratin 19, galectin 3, thyroperoxidase, and high-molecular-weight cy-tokeratin. All patients underwent CT before surgery, and a 3D slicer was used to analyze images of the surgical specimen. Test-retest and Spearman correlation coefficient (ρ) were used to select reproducible and nonredundant features. The Kruskal-Wallis test (p < 0.05) was used for featu-re selection, and a feature-based model was built by support vector machine methods. The per-formance of the radiomic models was assessed with respect to accuracy, sensitivity, specificity, corresponding AUC, and independent validation. RESULTS. Eighty-six reproducible and nonredun-dant features selected from the 828 features were used to build the model. The best performance of the cytokeratin 19 model yielded accuracy of 84.4% in the training cohort and 80.0% in the vali-dation cohort. The thyroperoxidase and galectin 3 predictive models yielded accuracies of 81.4% and 82.5% in the training cohort and 84.2% and 85.0% in the validation cohort. The performance of the high-molecular-weight cytokeratin predictive model was not good (accuracy, 65.7%) and could not be validated. CONCLUSION. A radiomics model with excellent performance was develo-ped for individualized noninvasive prediction of the presence of cytokeratin 19, galectin 3, and thy-roperoxidase based on CT images. This model may be used to identify benign and malignant thy-roid nodules. - Prevalence of Artificial Intelligence, HR Technology Research Team, 2020
This research will help HR leaders understand the prevalence of artificial intelligence in different regions as well as the most com-mon applications and the popularity of AI within HR - Radiomics in Pulmonary Lesion Imaging, Cameron Hassani, Bino A. Varghese, Jorge Nieva and Vinay Duddalwar
OBJECTIVE. Diagnostic imaging has traditionally relied on a limited set of qualitative imaging characteristics for the diagnosis and management of lung cancer. Radiomics—the extraction and analysis of quantitative features from imaging—can identify additional imaging characteristics that cannot be seen by the eye. These features can potentially be used to diagnose cancer, identify mutations, and predict pro-gnosis in an accurate and noninvasive fashion. This article provides insights about trends in ra-diomics of lung cancer and challenges to widespread adoption. CONCLUSION. Radiomic studies are currently limited to a small number of cancer types. Its application across various centers are nonstandardized, leading to difficulties in comparing and generalizing results. The tools available to apply radiomics are specialized and limited in scope, blunting widespread use and clinical inte-gration in the general population. Increasing the number of multicenter studies and consortiums and inclusion of radiomics in resident training will bring more attention and clarity to the growing field of radiomics. - Research Guide to Introducing Artificial Intelligence and Machine Learning in Legal Departments, Legal and Compliance Research Team, 2020
AI and ML can transform legal de-partments by automating manual tasks, creating insights and enabling better legal and complian-ce risk management. General counsel should use this note as a guide to the research that will chart their AI and ML adoption journey - Russia and NATO Artificial Intelligence in Military Market Detailed Analysis, Emerging Trends and Bussiness Opportunities (2020-2027), Milind, 2020
Facts and Factors (FnF), A leading market research firm published the latest report on “Russia and NATO Artificial Intelligence in Mi-litary Market By Application (Warfare Platform, Information Processing,Logistics & Transpor-tation, Target Recognition, Battlefield Healthcare, Simulation & Training, Threat Monitoring & Situational Awareness, Cybersecurity, and Others), by Platform(Land, Naval, Space, and Airborne), by Offering (Software, Hardware, and Services), and by Technology (Learning &Intelligence, AI Systems, and Advanced Computing): Industry Perspective, Comprehensive Analysis, and Forecast, 2019–2027” which includes 180+ research pages for the forecast period. The Russia and NATO Artificial Intelligence in Military market report offers comprehensive re-search updates and information related to market growth, demand, and opportunities in the glo-bal Russia and NATO Artificial Intelligence in Military market - Same father, same face: Deep learning reveals selection for signaling kinship in a wild primate
Using deep learning for face reco-gnition, we present the first evidence that interindividual facial resemblance has been selected to signal paternal … - Section Editor’s Notebook: Augmented Intelligence in Women’s Imaging—A Compelling Value Proposition, Marcia C. Javitt
Contemporary women’s imaging has evolved during the last few decades. Witness the changes brought about by the digital revolu-tion, such as the shift from film-screen mammography to 3D breast tomosynthesis and from cross-sectional imaging with 2D static images on hardcopy film to 3D volume datasets (e.g., 3D whole-breast ultrasound and volumetric breast MRI). The infinite display capabilities on advanced workstations at the discretion of the viewer are hallmarks of the digital era, as is functional ima-ging (e.g., PET/CT), molecular breast imaging (e.g., with 99mTc-sestamibi), and much more. Along with these technologic advancements has come a profound increase in the sheer volume of data associated with everyday examinations. The burden of analyzing huge datasets and compa-ring them with prior examinations falls squarely on the shoulders of the interpreting radiologist. The reality is that we are rapidly exceeding human capabilities to interpret this volume of data. Today’s radiologist must perform repetitive, accurate, and efficient pattern recognition and analy-sis. Though we strive for it, complete data extraction from complex, ever-increasing volumes of data is often infeasible with human interpretation. The popular term “artificial intelligence,” now heard far and wide in every department of radiology, should be dropped in favor of “augmented intelligence” (AI). The latter is a more apt descriptor of the power of modern supercomputers to expand and improve on human interpretation alone. AI entails the use of computers to perform pattern recognition by means of automatic feature extraction and analysis of large datasets much more rapidly than human readers can. AI includes deep learning through the use of algorithms with multiple layers (hence the term “deep”) to extract more complex features from training da-ta. High-volume and complex image analysis can be achieved with deep learning more rapidly than with conventional neural networks… - Seven Factors That Make Business Cases for Artificial Intelligence Projects Diffe-rent, Moutusi Sau, Whit Andrews, 2020
Application of artificial-intelligence-powered methods require more than just the latest technologies or modeling techni-ques. CIOs documenting business cases for AI projects should inspect the correlation between bu-siness benefits and challenges to achieve desired business outcomes - Should We Ignore, Follow, or Biopsy? Impact of Artificial Intelligence Decision Sup-port on Breast Ultrasound Lesion Assessment, Victoria L. Mango, Mary Sun, Ralph T. Wynn and Richard Ha, 2020
OBJECTIVE. The objective of this study was to assess the impact of artificial intelligence (AI)-based decision support (DS) on breast ultrasound (US) lesion assessment. MATERIALS AND METHODS. A multicenter retrospective revi-ew of 900 breast lesions (470/900 [52.2%] benign; 430/900 [47.8%] malignant) on US by 15 phy-sicians (11 radiologists, two surgeons, two obstetrician/gynecologists). An AI system (Koios DS for Breast, Koios Medical) evaluated images and assigned them to one of four categories: benign, probably benign, suspicious, and probably malignant. Each reader reviewed cases twice: 750 cases with US only or with US plus DS; 4 weeks later, cases were reviewed in the opposite format. One hundred fifty additional cases were presented identically in each session. DS and reader sensitivi-ty, specificity, and positive likelihood ratios (PLRs) were calculated as well as reader AUCs with and without DS. The Kendall τ-b correlation coefficient was used to assess intraand interreader variability. RESULTS. Mean reader AUC for cases reviewed with US only was 0.83 (95% CI, 0.78–0.89); for cases reviewed with US plus DS, mean AUC was 0.87 (95% CI, 0.84–0.90). PLR for the DS system was 1.98 (95% CI, 1.78–2.18) and was higher than the PLR for all readers but one. Fourteen readers had better AUC with US plus DS than with US only. Mean Kendall τ-b for US-only interreader variability was 0.54 (95% CI, 0.53–0.55); for US plus DS, it was 0.68 (95% CI, 0.67–0.69). Intrareader variability improved with DS; class switching (defined as crossing from BI-RADS category 3 to BI-RADS category 4A or above) occurred in 13.6% of cases with US only versus 10.8% of cases with US plus DS (p = 0.04). CONCLUSION. AI-based DS improves accuracy of sono-graphic breast lesion assessment while reducing inter- and intraobserver variability. - State of the Art: Machine Learning Applications in Glioma Imaging, Eyal Lotan, Ra-jan Jain, Narges Razavian, Girish M. Fatterpekar …
OBJECTIVE. Machine learning has recently gained considerable attention because of promising results for a wide range of radiology applications. Here we review recent work using machine learning in brain tumor imaging, specifi-cally segmentation and MRI radiomics of gliomas. CONCLUSION. We discuss available resources, state-of-the-art segmentation methods, and machine learning radiomics for glioma. We highlight the challenges of these techniques as well as the future potential in clinical diagnostics, progno-stics, and decision making. - Study: Network Representation Learning Could Help Identify MS-Related Genes, Ja-red Kaltwasser, 2020
New research suggests deep lear-ning algorithms and computational methods could help scientists better understand the mecha-nisms at play in multiple sclerosis (MS). Scientists know a lot about MS, but one question that has yet to be solved is which specific genes are related to the disease. In a new study, published in the journal Frontiers in Genetics,1 investigators suggest a type of computational network analysis might be the best pathway to discover the exact disease-related genes of MS. MS disrupts a pa-tient’s myelin and axons, leading to inflammation of the brain and spinal cord. And while some evidence has suggested certain disease-related genes that may play a role in MS2, the unknowns still outweigh the knowns, according to corresponding author Haijie Liu, PhD, of Capital Medical University and Tianjin Medical University General Hospital, both in China. Liu and colleagues say the discovery of MS’s disease-related genes could have major implications for how scientists un-derstand and how clinicians eventually treat patients with the disease… - The Doctor-Patient Relationship With Artificial Intelligence, Shadi Aminololama-Shakeri1 and Javier E. López
OBJECTIVE. The doctor-patient re-lationship has been evolving from benevolent paternalism to a more patient-centered relationship in the modern era. Although artificial intelligence (AI) has the potential to improve nearly every aspect of health care, many physicians are skeptical about integrating AI into their current medi-cal practice. The purpose of this article is to explore what AI means for the doctor-patient rela-tionship and for breast imaging radiologists. CONCLUSION. The promise of AI is its potential to re-lease physicians from tasks that are better performed by automation. AI may enhance our diagno-stic accuracy to the point that we are able to refocus on the art of the doctor-patient relation-ship. - The Future of Data Science, Machine Learning and AI, Peter Kerensky, 2020
Discussion Topics:Current and emerging trends to understand in data science and machine learning; How the future of AI should shape your strategy today; The coming challenges and opportunities around augmented analytics and MLOps. Artificial intelligence (AI) adoption has entered the mainstream, but most organiza-tions remain in the early stages, developing strategy and governance. Get a sense of where you stand, where things are headed and plan what is next for your data science professionals and expanding machine learning (ML) initiatives. In this complimentary webinar, learn where to invest energy and resources now to better capitalize on the technology landscape of the new deca-de - Three-Dimensional Convolutional Neural Network for Prostate MRI Segmentation and Comparison of Prostate Volume Measurements by Use of Artificial Neural Network and Ellipsoid Formula, Dong Kyu Lee, Deuk Jae Sung, Chang-Su Kim, Yuk Heo …
OBJECTIVE. The purposes of this study were to assess the performance of a 3D convolutional neural network (CNN) for automatic segmentation of prostates on MR images and to compare the volume estimates from the 3D CNN with those of the ellipsoid formula. MATERIALS AND METHODS. The study included 330 MR ima-ge sets that were divided into 260 training sets and 70 test sets for automated segmentation of the entire prostate. Among these, 162 training sets and 50 test sets were used for transition zone segmentation. Assisted by manual segmentation by two radiologists, the following values were obtained: estimates of ground-truth volume (VGT), software-derived volume (VSW), mean of VGT and VSW (VAV), and automatically generated volume from the 3D CNN (VNET). These values were compared with the volume calculated with the ellipsoid formula (VEL). RESULTS. The Dice similarity coefficient for the entire prostate was 87.12% and for the transition zone was 76.48%. There was no significant difference between VNET and VAV (p = 0.689) in the test sets of the en-tire prostate, whereas a significant difference was found between VEL and VAV (p < 0.001). No significant difference was found among the volume estimates in the test sets of the transition zone. Overall intraclass correlation coefficients between the volume estimates were excellent (0.887–0.995). In the test sets of entire prostate, the mean error between VGT and VNET (2.5) was smaller than that between VGT and VEL (3.3). CONCLUSION. The fully automated network studied provides reliable volume estimates of the entire prostate compared with those obtained with the ellipsoid formula. Fast and accurate volume measurement by use of the 3D CNN may help clinicians evaluate prostate disease. - Toolkit: Artificial Intelligence Use Cases for Insurance, Kimberly Harris-Ferrante, Manav Sachdeva, 2020
Insurers mentioned AI as the top “game-changing” technology in the 2020 Gartner CIO Survey. This customizable Toolkit enables CIOs to identify use cases for this advanced technology across the value chain to help realize the potential that it holds in driving business success - Unenhanced CT Texture Analysis of Clear Cell Renal Cell Carcinomas: A Machine Learning–Based Study for Predicting Histopathologic Nuclear Grade, Burak Kocak, Emine Sebnem Durmaz, Ece Ates, Ozlem Korkmaz Kaya …
OBJECTIVE. The purpose of this study is to investigate the predictive performance of machine learning (ML)–based unenhanced CT texture analysis in distinguishing low (grades I and II) and high (grades III and IV) nuclear grade clear cell renal cell carcinomas (RCCs). MATERIALS AND METHODS. For this retrospective study, 81 patients with clear cell RCC (56 high and 25 low nuclear grade) were included from a public da-tabase. Using 2D manual segmentation, 744 texture features were extracted from unenhanced CT images. Dimension reduction was done in three consecutive steps: reproducibility analysis by two radiologists, collinearity analysis, and feature selection. Models were created using artificial neu-ral network (ANN) and binary logistic regression, with and without synthetic minority oversam-pling technique (SMOTE), and were validated using 10-fold cross-validation. The reference stan-dard was histopathologic nuclear grade (low vs high). RESULTS. Dimension reduction steps yiel-ded five texture features for the ANN and six for the logistic regression algorithm. None of clinical variables was selected. ANN alone and ANN with SMOTE correctly classified 81.5% and 70.5%, respectively, of clear cell RCCs, with AUC values of 0.714 and 0.702, respectively. The logistic re-gression algorithm alone and with SMOTE correctly classified 75.3% and 62.5%, respectively, of the tumors, with AUC values of 0.656 and 0.666, respectively. The ANN performed better than the logistic regression (p < 0.05). No statistically significant difference was present between the model performances created with and without SMOTE (p > 0.05). CONCLUSION. ML-based unenhanced CT texture analysis using ANN can be a promising noninvasive method in predicting the nuclear grade of clear cell RCCs. - Universal Language Model Fine-tuning for Text Classi-fication, 2018
Inductive transfer learning has greatly impacted computer vision, but existing approaches in NLP still require task-specific modi-fications and training from scratch. We propose Universal Language Model Fine-tuning (ULMFiT), an effective transfer learning method that can be applied to any task in NLP, and introduce tech-niques that are key for fine-tuning a language model. Our method significantly outperforms the state-of-the-art on six text classification tasks, reducing the error by 18-24% on the majority of datasets. Furthermore, with only 100 labeled examples, it matches the performance of training from scratch on 100x more data. We open-source our pretrained models and code - Use of Gradient Boosting Machine Learning to Predict Patient Outcome in Acute Ischemic Stroke on the Basis of Imaging, Demographic, and Clinical Information, Yuan Xie, Bin Jiang, Enhao Gong, Ying Li …
OBJECTIVE. When treatment deci-sions are being made for patients with acute ischemic stroke, timely and accurate outcome pre-diction plays an important role. The optimal rehabilitation strategy also relies on long-term out-come predictions. The decision-making process involves numerous biomarkers including imaging features and demographic information. The objective of this study was to integrate common stro-ke biomarkers using machine learning methods and predict patient recovery outcome at 90 days. MATERIALS AND METHODS. A total of 512 patients were enrolled in this retrospective study. Ex-treme gradient boosting (XGB) and gradient boosting machine (GBM) models were used to pre-dict modified Rankin scale (mRS) scores at 90 days using biomarkers available at admission and 24 hours. Feature selections were performed using a greedy algorithm. Fivefold cross validation was applied to estimate model performance. RESULTS. For binary prediction of an mRS score of greater than 2 using biomarkers available at admission, XGB and GBM had an AUC of 0.746 and 0.748, respectively. Adding the National Institutes of Health Stroke Score at 24 hours and perfor-ming feature selection improved the AUC of XGB to 0.884 and the AUC of GBM to 0.877. With the addition of the recanalization outcome, XGB’s AUC improved to 0.807 for nonrecanalized patients and dropped to 0.670 for recanalized patients. GBM’s AUC improved to 0.781 for nonrecanalized patients and dropped to 0.655 for recanalized patients. CONCLUSION. Decision tree–based GBMs can predict the recovery outcome of stroke patients at admission with a high AUC. Breaking down the patient groups on the basis of recanalization and nonrecanalization can potentially help with the treatment decision process. - Utility of CT Radiomics Features in Differentiation of Pancreatic Ductal Adenocarci-noma From Normal Pancreatic, Tissue Linda C. Chu, Seyoun Park, Satomi Kawamoto, Daniel F. Fouladi …
OBJECTIVE. The objective of our study was to determine the utility of radiomics features in differentiating CT cases of pancreatic ductal adenocarcinoma (PDAC) from normal pancreas. MATERIALS AND METHODS. In this retro-spective case-control study, 190 patients with PDAC (97 men, 93 women; mean age ± SD, 66 ± 9 years) from 2012 to 2017 and 190 healthy potential renal donors (96 men, 94 women; mean age ± SD, 52 ± 8 years) without known pancreatic disease from 2005 to 2009 were identified from ra-diology and pathology databases. The 3D volume of the pancreas was manually segmented from the preoperative CT scans by four trained researchers and verified by three abdominal radiologi-sts. Four hundred seventy-eight radiomics features were extracted to express the phenotype of the pancreas. Forty features were selected for analysis because of redundancy of computed featu-res. The dataset was divided into 255 training cases (125 normal control cases and 130 PDAC ca-ses) and 125 validation cases (65 normal control cases and 60 PDAC cases). A random forest clas-sifier was used for binary classification of PDAC versus normal pancreas of control cases. Accura-cy, sensitivity, and specificity were calculated. RESULTS. Mean tumor size was 4.1 ± 1.7 (SD) cm. The overall accuracy of the random forest binary classification was 99.2% (124/125), and AUC was 99.9%. All PDAC cases (60/60) were correctly classified. One case from a renal donor was misclassified as PDAC (1/65). The sensitivity was 100%, and specificity was 98.5%. CONCLUSION. Radiomics features extracted from whole pancreas can be used to differentiate between CT cases from patients with PDAC and healthy control subjects with normal pancreas. - Value of Texture Analysis on Gadoxetic Acid–Enhanced MRI for Differentiating Hepatocellular Adenoma From Focal Nodular Hyperplasia, Roberto Cannella, Balasubramanya Rangaswamy, Marta I. Minervini, Amir A. Borhani …
OBJECTIVE. The objective of our study was to assess the diagnostic performance of texture analysis (TA) on gadoxetic acid–enhanced MR images for differentiation of hepatocellular adenoma (HCA) from focal nodular hy-perplasia (FNH). MATERIALS AND METHODS. This study included 40 patients (39 women and one man) with 51 HCAs and 28 patients (27 women and one man) with 32 FNH lesions. All lesions were histologically proven with preoperative MRI performed with gadoxetic acid. Two readers re-viewed all the imaging sequences to assess the qualitative MRI characteristics. The T2-weighted fast spin-echo, hepatic arterial phase (HAP), and hepatobiliary phase (HBP) sequences were used for TA. Textural features were extracted using commercially available software (TexRAD). The dif-ferences in distributions of TA parameters of FNHs and HCAs were assessed using the Mann-Whitney U test. Area under the ROC curve (AUROC) values were calculated for statistically signifi-cant features. A logistic regression analysis was conducted to explore the added value of TA. A p value < 0.002 was considered statistically significant after Bonferroni correction for multiple comparisons. RESULTS. Multiple TA parameters showed a statistically different distribution in HCA and FNH including skewness on T2-weighted imaging, skewness on HAP imaging, skewness on HBP imaging, and entropy on HBP imaging (p < 0.001). Skewness on HBP imaging showed the largest AUROC (0.869; 95% CI, 0.777–0.933). A skewness value on HBP imaging of greater than −0.06 had a sensitivity of 72.5% and a specificity of 90.6% for the diagnosis of HCA. Six of 51 (11.8%) HCAs lacked hypointensity on HBP imaging. A binary logistic regression analysis including hypointensity on HBP imaging and the statistically significant TA parameters yielded an AUROC of 0.979 for the diagnosis of HCA and correctly predicted 96.4% of the lesions. CONCLUSION. TA may be of added value for the diagnosis of atypical HCA presenting without hypointensity on HBP ima-ging. - What Does Deep Learning See? Insights From a Classifier Trained to Predict Contrast Enhancement Phase From CT Images, Kenneth A. Philbrick, Kotaro Yoshida, Dai Inoue, Zeynettin Akkus …
OBJECTIVE. Deep learning has shown great promise for improving medical image classification tasks. However, knowing what aspects of an image the deep learning system uses or, in a manner of speaking, sees to make its prediction is difficult. MATERIALS AND METHODS. Within a radiologic imaging context, we inve-stigated the utility of methods designed to identify features within images on which deep lear-ning activates. In this study, we developed a classifier to identify contrast enhancement phase from whole-slice CT data. We then used this classifier as an easily interpretable system to explore the utility of class activation map (CAMs), gradient-weighted class activation maps (Grad-CAMs), saliency maps, guided backpropagation maps, and the saliency activation map, a novel map repor-ted here, to identify image features the model used when performing prediction. RESULTS. All techniques identified voxels within imaging that the classifier used. SAMs had greater specificity than did guided backpropagation maps, CAMs, and Grad-CAMs at identifying voxels within ima-ging that the model used to perform prediction. At shallow network layers, SAMs had greater specificity than Grad-CAMs at identifying input voxels that the layers within the model used to perform prediction. CONCLUSION. As a whole, voxel-level visualizations and visualizations of the imaging features that activate shallow network layers are powerful techniques to identify fea-tures that deep learning models use when performing prediction - What’s Trending With CIOs: The Crunch for AI Talent, Peter Kerensky, 2020
High demand and tight labor mar-kets have made candidates with AI skills highly competitive, but hiring techniques and strategies have not kept up. This research discusses what’s trending with CIOs regarding AI talent
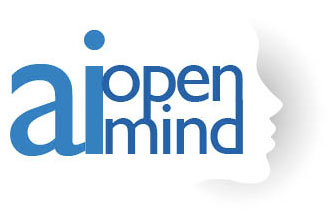
Insieme per riflettere sull’intelligenza - umana e artificiale - tra studi, esperienze, democrazia e innovazione.